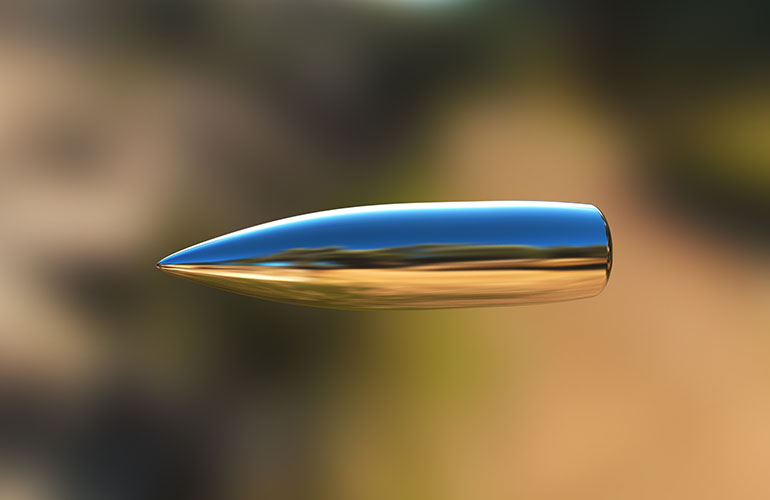
[Image courtesy of Scanrail/Adobe Stock]
Imagine a world where the process of developing life-saving drugs is as streamlined as a modern assembly line.
In such a reality, generative AI in drug discovery might churn out promising compounds with similar efficiency and precision as a factory robot assembling a car. Moreover, such technology could chip away at the steep cost and lengthy timelines typically associated with drug development, which can cost north of $2 billion and take more than a decade.
However, such a vision may be more hype than reality, according to a new UBS report. While it is true that AI is carving out a niche in life sciences, a recent Q-Series report from UBS titled “Will Generative AI deliver a generational transformation?” reaches mostly muted conclusions about the potential of generative AI in drug development. In essence, the investment bank projects that generative AI will reduce pharma costs, but the technology is unlikely to have an appreciable impact on growth or the competitive landscape.
In essence, UBS deems generative AI in biopharma to be more of an evolution than a revolution. That is, it could assist in areas such as protein mapping and compound screening, but generative AI may not lead to an R&D renaissance in the foreseeable future.
Gauging the potential of generative AI in drug discovery and development
All of this is not to say UBS is writing off generative AI in drug discovery and development. On the contrary, its report points out that the technology could expedite processes and trim the enormous costs involved in traditional drug development. That is, generative AI could be “a potential part of the toolbox,” according to UBS.
The investment bank notes that it can accelerate protein mapping, including ligand identification, and play a role in IP infringement prevention. It can also assist with compound screening. But it adds a caveat, noting “whether this leads to more/better drugs is unclear.”
Data quality questions persist in biopharma
Navigating the complex data landscape in biopharma poses significant challenges and opportunities. Though the industry might appear data-rich on the surface, fully comprehending the benefit-risk ratio for even a single drug can prove challenging. For one thing, head-to-head clinical trials are relatively uncommon. And when data from head-to-head trials is available, there often remains a lack of evidence that would clearly support direct comparisons of the efficacy or safety of one drug versus another.
In addition, data availability is another common hurdle in biopharma. For one thing, the industry has not historically reported negative trial results as comprehensively as positive ones, leading to a skewed dataset. Added to this are the realities that much drug data is proprietary and data related to drug development data are often patchy. While some of the best available data relates to toxicity, UBS notes that even this is fraught with complexities as toxicity isn’t necessarily compound-specific. Factors such as dosage, genetics and patient characteristics, including age, play vital roles in determining toxicity.
Another consideration is that the known universe of studied drug compounds is limited. UBS puts the number of drug compounds administered to humans in the ballpark of 10,000. That figure is “not many” in “the bigger scheme of things, and in the context of computational chemistry,” UBS adds.
“Data quality and data representation are real challenges, making [generative AI] more evolution than revolution,” the investment bank’s report concluded.
This situation aligns with the well-known software aphorism “garbage in, garbage out” (GIGO), implying that the input quality determines the output quality. In other words, incomplete or biased input data tends to lead to flawed or limited insights.
Data representation challenges are another consideration
Another obstacle lies in the representation of data. In drug development, the best representation of chemical or biological data is often unclear. The UBS report points out that 2D representations of molecules do not adequately capture the complexities of chemical and biological data. This reality can prove to be a challenge when encoding such data in, say, machine learning, which depends heavily on statistical pattern recognition. Given the multi-dimensional nature of molecular data, such 2D representations can result in missing context, limiting the insights of machine learning techniques.
Exploring the long-term potential of generative AI in pharma
Despite such complexities, the potential of generative AI to drive progress in biopharma is considerable. The UBS report suggests that generative AI can help with patient selection or biomarker selection for clinical development.
The report quotes IT analyst Gartner, which projects that generative AI techniques will play a role in discovering 30% of new drugs and materials by 2025. It also cites Accenture, which estimates that 40% of all working hours (not just in pharma) could be impacted, ranging from 9% to 63% of the typical workday.
Generative AI could also potentially help the industry reduce its reliance on animal testing, mitigating a significant ESG risk.
But UBS also notes that the technology may reduce potential market sizes. That is, the technology could assist in the development of more targeted, personalized treatments for narrower populations. Yet the report also suggests that AI can open up new markets by supporting the development of therapies for previously untreatable or poorly understood conditions, creating new markets. Next, the ability of AI to personalize treatments could lead to new market segments in existing markets. Finally, AI could drive greater efficiency in drug discovery and development, making it economically feasible to target diseases that affect smaller patient populations than with traditional methods.
From drug discovery to broader economic impacts: The generative AI evolution
In the long run, the economic and societal implications of generative AI reach far beyond drug discovery and development. As an April Goldman Sachs Research report noted, generative AI could drive sweeping changes to the global economy. The firm predicts that generative AI could drive a 7% increase in global GDP and lift productivity growth by 1.5% points over a 10-year period. It also notes that AI could partially automate two-thirds of occupations.
While generative AI might not usher in a renaissance in drug discovery overnight, drug developers that learn how to harness the technology will likely find themselves at a competitive advantage. Assuming that generative AI will offer a more evolutionary advances to drug developers, the technology could play a role in a survival of the fittest dynamic. Drug developers that can use AI technologies to adapt and reimagine their business could potentially spark progress that reaches well beyond the lab and into the heart of global healthcare.