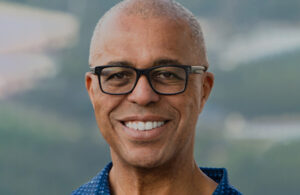
Medtronic SVP and Chief Technology and Innovation Officer Ken Washington [Photo courtesy of Medtronic]
Medtronic SVP and Chief Technology and Innovation Officer Ken Washington was recently briefing the 15 general managers who run each of the operating units at the world’s largest medical device manufacturer.
In the middle of the first chart in his presentation on artificial intelligence, one of the leaders stopped him.
As Washington tells it, they said, “I just don’t understand all these different buzzwords around AI. Can you tell me what are the different types of AI? How does it all work? And what’s the difference between generative AI and deep learning?”
Washington — who joined Medtronic in June 2023 after serving as VP and GM of consumer robotics at Amazon and CTO at Ford Motor Co. before that — pulled out an easel, grabbed a marker, and walked the group through the basics.
In an interview with Medical Design & Outsourcing, Washington offered an abbreviated version of that tutorial. The following quotes have been lightly edited for space and clarity.
“What we’ve got to do as leaders is to teach our business leaders — those who don’t practice this every day, who haven’t been in the position of running a robot program like I have, or haven’t done things like what Medtronic Endoscopy Chief AI Officer Ha Hong has done — what these terms mean, why it matters, how it’s evolving, what it’s good for and what it’s not good for, what’s hype, what’s real, raising our fluency. And then we can apply this in a strategic way that matters.
“AI as a term was first coined in 1956. It’s the math and science of emulating human decisions on a computer. And the first iterations of that were done with rule-based systems. There are some logic machines that were very purpose-built for doing that. None of them were very good. They couldn’t solve problems at any large appreciable scale, they weren’t very general-purpose. And so the field stagnated for a long time.
“And then machine learning burst on the scene. That’s the first specialization that happened. This is the science of teaching the machine how to make decisions by creating digital neurons and connecting them with weights. The weights are actually established by teaching the neurons how they interact with each other by feeding it data. The more data you feed it, the better the weights are, and the better the neurons are able to interact with each other to take an input and give you an output that actually makes sense. And that worked pretty well.
“But the number of layers were limited, because it was too expensive computationally to actually assign the weights and run the algorithms to give you the output until Geoffrey Hinton came along. He and his fast followers figured out a way to add many different additional layers to those networks, and that created what’s called deep neural networks or deep learning. That allowed us to build models that had many layers and many digital neurons connected by these weights that are trained on massive amounts of data. They had all kinds of algorithms and methods for doing backpropagation and forward propagation that made the ability to do deep neural networks computationally feasible. At the same time, the amount of data that was available to train these networks went through an exponential explosion — and the computers to run these models got so much faster, graphics processing units (GPUs) got much better. NVIDIA burst on the scene with their big special-purpose GPUs to do these computations. So the confluence of Big Data, GPUs, and these new algorithms for doing deep learning forward and backward propagation made deep learning really feasible. That, for the first time, made artificial intelligence in some cases better than humans, and in many cases almost as good as humans at doing image recognition, speech recognition and solving other problems.
“Then, some smart guys and gals at Google came up with these Transformer models. That allowed them to build not just deep models, but massive multibillion-parameter models that were trained by massive data sets and that’s what created foundational models and generative AI, which not only is able to do image and voice recognition quite well, but it can generate new content based on what it learned in the past that’s realistic looking, sounding and seeming. Generative AI is a very specialized field of deep learning that uses these big, huge foundation models that have to be trained on these massive parallel supercomputers that these big tech companies had access to. That’s what burst onto the scene in about 2017. And the reason it only became pop culture-like — an explosion of scale and hype and excitement in November of last year — was because OpenAI packaged that in a consumer-facing tool and put it on the web in chat: ChatGPT. And the rest is history.
“The sweet spot in medtech is deep learning. It’s got to be good enough to be able to aid a clinician to make a decision or to be informed or advised. Deep learning is really good at that — you don’t need generative AI. In fact, generative AI has all kinds of side effects that are not really well suited to medtech, like hallucination.
“We have to remind ourselves that while AI technology has been around for a long time, the modern-day version of AI — with these big foundation models, or even these AI solutions that are built on these deep neural networks that have very sophisticated algorithms for training the networks, running on some of the world’s most complex cloud supercomputers and some on-prem supercomputers — has only been with us for one to two years. And so we have to spend the time and take the effort to teach ourselves and to teach others about the importance of these technologies.
“The future is bright, and we’re just getting started.”