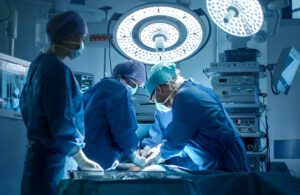
Improving the health of high-risk patients before their surgeries can lower mortality rates and cut healthcare costs. [Image by Gorodenkoff via Adobe Stock]
A newly developed machine learning model for surgical patients is automatically flagging those at high risk of complications to improve their odds of survival and reduce healthcare system costs.
Each day, the software reviews electronic medical records for patients scheduled for surgery and identifies those who might benefit from individualized coordinated care or prehabilitation to improve surgical results.
Researchers and physicians at the University of Pittsburgh and University of Pittsburgh Medical Center (UPMC) trained their algorithm on medical records for more than 1.2 million surgical patients. To help predict whether patients might suffer from complications after surgery, they focused the model on deaths from strokes, heart attacks and other major cerebral or cardiac events.
The researchers said their model did a better job of identifying high-risk patients than the American College of Surgeon’s National Surgical Quality Improvement Program (ACS NSQIP) used by hospitals nationwide, which requires manual data entry.
Surgical complications are a leading killer
Surgical complications within 30 days of a procedure kill an estimated 4.2 million people globally per year. Surgical complications were the third leading contributor of death in the U.S. before the COVID-19 pandemic.
Up to 15% of patients may experience complications within 30 days of an operation, costing hospitals more than $11,000 per case and more than $31.3 billion nationally per year, the researchers said.
High-risk patients make up about half of the 15% of patients who experience surgical complications. Improving the health of those high-risk patients before their surgeries can lower mortality rates and cut healthcare costs — if doctors know who’s most likely to benefit.
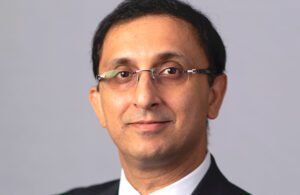
Dr. Aman Mahajan [Photo courtesy of UPMC]
The teams were led by Dr. Aman Mahajan, chair of Anesthesiology and Perioperative Medicine at Pitt School of Medicine and director of UPMC Perioperative and Surgical Services, and Dr. Oscar Marroquin, chief health care data and analytics officer at UPMC.
“Improving overall health of patients prior to surgery through prehabilitation can go a long way in improving outcomes for high-risk patients,” Mahajan said in a news release. “However, identifying high-risk patients can be challenging for busy clinicians, who have to integrate the wealth of health data available and frequently perform additional testing and clinical assessments. We wanted to build an easy-to-use model that provides the health care team with an automated and accurate risk assessment quickly using existing data in the electronic health record.”
After training the algorithm, the teams validated the model against 200,000 UPMC surgical patients and rolled it out across 20 UPMC hospitals.
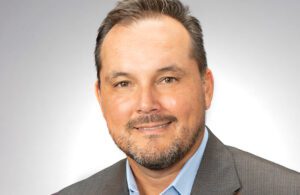
Dr. Oscar Marroquin [Photo courtesy of UPMC]
“We designed our model with the health care worker in mind,” Marroquin said. “Since our model is completely automated and can make educated predictions even if some data are missing, it adds almost no additional burden to clinicians while providing them a reliable and useful tool.”
In addition to the daily, automated reviews, clinicians can run the model anytime on-demand.
The researchers continue to improve the software, with plans to add the ability to predict the likelihood of sepsis, respiratory issues and other complications that keep patients hospitalized after surgery.
More details are available in the UPMC news release and the study, published in JAMA Network Open.
Related: Artificial intelligence breakthroughs in medtech: 7 ways to enhance healthcare