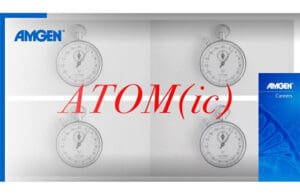
[Image credit: Amgen]
Amgen has developed a machine learning platform to slash clinical trial times through smarter site selection. Known as ATOMIC, short for Analytical Trial Optimization Module, the system crunches disparate datasets to predict optimal trial locations, expedite enrollment and trial processes. Early results indicate more than a two times increase in enrollment speed at ATOMIC sites.
ML-powered clinical trial oracle could compress clinical trial cycle time
“With the massive amounts of data we’re pulling from various sources, we anticipate that by 2030, we’ll be able to shave about two years off the development times for our drugs,” said Sheryl Jacobs, vice president, global Development operations at Amgen. For now, the company is steadily ramping up the number of trials using the ATOMIC process. “Within the next year or two, we expect the majority of our trials will be using the AI and machine learning approach,” Jacobs said.
ATOMIC is the brainchild of Amgen data scientists, engineers and analysts who aimed to transform site selection through advanced data modeling. Using open-source Python tools, the cross-functional team developed an engine that crunches large data volumes to predict optimal trial locations based on efficient patient enrollment. This initiative reflects Amgen’s broader strategy of tapping advanced technologies in drug development, as seen in its collaboration with NVIDIA to accelerate biologics discovery.
In a video released last year, Amgen described ATOMIC as its largest, most complex data science project to date, involving extensive global clinical trial sites and diverse datasets. The data ATOMIC parses contains a vast array of potential features to analyze, drawing connections that would have been impossible for clinical trial professionals to infer alone.
The inside story of ATOMIC’s development
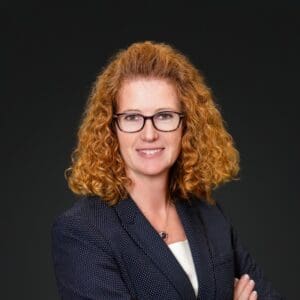
Sheryl Jacobs
Amgen developed ATOMIC largely internally through a cross-functional team that created algorithms to synthesize both internal and public data sources. The goal was to focus on investigators most likely to excel and enroll patients quickly based on past performance.
“ATOMIC allows us to make predictions in an informed way and build a ranked list of sites that may perform best,” Jacobs said. This was a significant shift from the traditional manual, time-consuming process of site selection.
In creating ATOMIC, Jacobs emphasized the critical role of interdisciplinary collaboration, highlighting the need for “folks with operational expertise to translate for the technical team.” She stressed the importance of combining “deep clinical operations expertise with technical data scientists” for the project’s success. Jacobs noted that ATOMIC’s success is a result of professionals adept at translating clinical needs into technical data science applications.
Collaboration and feedback are also central in ATOMIC. Hypotheses are continuously generated, tested and refined across teams to enhance decision-making. Early in its implementation, ATOMIC has already identified clinical trial sites that may have otherwise been overlooked using traditional methods.
The need to blend operational expertise and technical acumen
Professionals who can translate between clinical and technical domains are vital for ATOMIC’s success. Combining operations expertise with data science skills enables effective collaboration. Skilled translators, grasping both clinical and technical domains, can guide data scientists on the most meaningful analytic factors based on the clinical teams’ needs. They can also translate the technical output into actionable insights for the clinical trial managers. This cross-functional translation ability is still relatively uncommon, but is proving essential as data science and AI transform operations. Ultimately, ATOMIC’s approach shows the power of close collaboration between technical and operational experts in accelerating innovation.”
By integrating disparate data sources like anonymized real-world data from electronic health records and medical claims, ATOMIC provides a single, comprehensive information source to streamline site selection. The system helps identify key drivers of enrollment, leading to better predictions of the most successful sites.
“A lot of cross-functional planning and partnering goes into making a clinical trial successful,” Jacobs said. “ATOMIC allows us to streamline that process.”
Diversity by design
Integrating real-world data into ATOMIC also optimizes the diversity of clinical trial populations. By mirroring real-world demographic data on disease prevalence, the framework helps ensure recruited populations represent the diversity of patients affected by the disease.
The RISE (Representation in Clinical Research) diversity scorecard allows assessment of the diversity of potential trial populations at specific sites. “While it doesn’t dictate the number of patients we can get, it does provide an indicator of whether the site has a diverse population we could potentially recruit from.”
The AI/ML approach generates a diversity scorecard for potential sites, enabling assessment of available diverse populations, Jacobs noted. The scorecard is “absolutely critical for our clinical trials moving forward,” Jacobs said. “It allows us to evaluate the potential diverse populations that could be enrolling in our trials, based on the sites selected through AI and machine learning.”