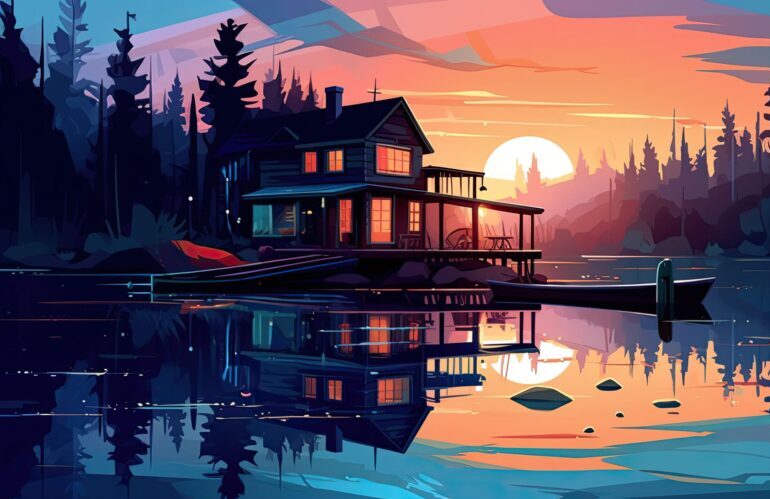
[Outlier Artifacts/Adobe Stock]
In recent years, the term “data lakehouse” has entered the lexicon of data professionals. For AI-enabled clinical trials, the lakehouse architecture promises seamless integration of diverse data streams, spanning patient health records to real-time sensor data, all processed efficiently and queried in structured formats.
The lakehouse architecture aims to provide a comprehensive overview of data, ensuring both vast storage and real-time processing capabilities. In other words, the lakehouse offers the “best of both worlds” when it comes to data warehouses and data lakes, according to Venu Mallarapu, vice president of global strategy and operations at eClinical Solutions.
AI and ML move from buzzwords to practical tools in clinical trial management
As the use of AI and ML in clinical trials becomes more prevalent in patient recruitment, real-time data monitoring and beyond, the lakehouse architecture could provide a seamless and integrated data management option. That’s because it can bridge the gap between the structured querying capabilities and performance of a data warehouse and the scalable storage and flexibility of a data lake. Notably, the application of AI and ML in data management within the lakehouse architecture can significantly bolster clinical trial efficiency. In addition, the ability to source data directly from electronic health records (EHRs) and electronic medical records (EMRs) can bypass the need for traditional electronic data capture (EDC) systems, further streamlining the data management process.
In addition, the increasing use of wearable sensors in clinical trials, can generate rich time-series data that a lakehouse environment can help manage and interpret. Despite the promise of the data lakehouse approach, adoption remains at an early stage. Migrating existing on-premise databases to the new architecture is one potential barrier, given the technical and change management challenges that can arise when adopting a new architecture. Another factor is the inertia of well-established traditional data warehouses, which have been foundational in enterprise data management for decades.
Data warehouse: Traditional strengths and weaknesses
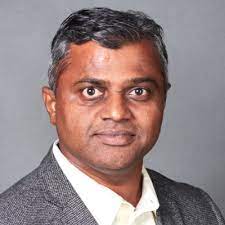
Venu Mallarapu,
Between data warehouses and data lakes, data warehouses have been around much longer. The genesis of the data warehouse concept dates back to the 1980s and early 1990s, when the computer scientist and prolific author Bill Inmon popularized the concept. The idea was to centralize data traditionally stored in silos, offering a horizontal view of organizational information. The architecture has since cemented its status as a foundational component in enterprise data management. “That architecture is quite conducive for structured data and data that is in rows and tables,” Mallarupu said.
Traditionally, they have excelled at extracting data from transactional systems or systems of record, transforming the data into a chosen format, and loading it into a repository. This process is often referred to as “ETL,” an abbreviation of extract, transform, load.
At present, many organizations focused on clinical trials continue to rely on the data warehouse approach. But as the clinical trial landscape evolves thanks to the rising reliance on decentralized clinical trials, omics data, and other advanced scientific methods, the data warehouse approach is less ideal. “That’s where the need for the lake house is coming into play,” Mallarupu said.
The rise of data lakes
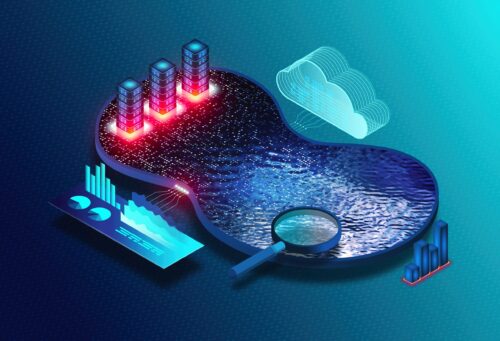
[ArtemisDiana/Adobe Stock]
The data lake first became popular after James Dixon, then the chief technology officer of the business intelligence firm Pentaho, coined the term in 2010 to refer to vast repositories of raw data. “Data lakes came along with the advent of the cloud and cheaper storage,” Mallarupu recalled.
Data lakes can store large volumes of raw data, both structured and unstructured. The idea is that a data lake can hold vast amounts of data in its natural, raw state. Data lakes allow users to perform traditional ETL processes on structured data but also extract information from unstructured data. Such unstructured sources can include text, images, audio, and video that lack a predefined data model.
Compared to data warehouses, data lakes offer considerably more flexibility as they can ingest data in real-time from an array of sources without the need for an immediate structure or schema. In recent years, pharma companies such as Bristol-Myers Squibb (BMS), Takeda Pharmaceutical, and Amgen have implemented data lakes to boost the speed and efficiency of their research processes. But data lakes can be difficult to configure. The architecture can prove challenging when it comes to maintaining data integrity. And without proper governance, a data lake can degrade into a data swamp. Despite their flexibility, it takes work to maintain data integrity and quality in a data lake.
Enter the data lakehouse architecture: A powerful foundation for AI
As mentioned at the outset, a lakehouse is a hybrid approach that offers the best of both worlds. The environment is a good fit for data-hungry AI/ML projects, Mallarupu said. The environment offers a repository that can store data, whether it is structured, unstructured, or even semi structured. And in contrast to a data lake, the lakehouse offers the structured querying and data management capabilities of data warehouses.
For AI-enabled clinical trials, the lakehouse architecture unifies diverse data streams, from patient health records to real-time sensor data, all processed efficiently and queried in structured formats. By providing a comprehensive overview of data, including vast storage and real-time processing capabilities, the data lakehouse can support data scientists’ need. “You can draw the data that you need for AI training, testing, and validating the AI/ML models directly,” Mallarupu said.
In addition, lakehouse architecture has the potential to support the application of generative AI (gen AI), given that it can handle diverse data types and ensure data privacy.
As AI/ML tools emerge to address specific needs in clinical research, they promise to boost the efficiency and accuracy of various aspects of clinical trials, spanning patient recruitment, data analysis and decision-making. But implementing AI/ML in a highly regulated environment is not without challenges. One of the main hurdles is finding ways to tap such powerful tools while maintaining data privacy and complying with stringent regulations. The lakehouse architecture, with its robust security measures and compliance features, can help address these challenges by providing a secure and compliant environment for data processing and analysis.
Burgeoning support for the data lakehouse architecture in clinical trials
eClinical Solutions uses the architecture in its platform, elluminate, to help pharma R&D professionals with decision-making in clinical trials. More than 100 life science organizations are using the platform, including Bristol Myers Squibb, bluebird bio, Jounce Therapeutics, Agios, and Urovant Sciences.
In the broader landscape, other companies have adopted the data lakehouse for clinical data management and analytics, such as Amgen and Verana Health.
The data lakehouse architecture supports real-time data monitoring, which, for clinical trial data, is important as it can detect any anomalies that could indicate safety problems, allowing for immediate action to be taken to address any potential risks to patient safety. While a data warehouse could potentially support real-time data monitoring, a data lakehouse is more efficient in this regard, given the architecture’s ability to seamlessly integrate diverse data streams, handle vast volumes of structured and unstructured data, and provide both scalable storage and agile querying capabilities.
Organizations using the architecture can also develop purpose-built applications or products while also furthering AI initiatives. Companies that succeed in tapping AI and ML to drive decisions, whether operational or scientific, will have “a huge advantage,” Mallarapu said. In this context, the data lakehouse architecture provides a solid foundation that “not only meets your needs today but is future-proof,” he concluded.