
[Egor/Adobe Stock]
In drug discovery, the process of shooting for regulatory approval can feel less like a sprint and more like a marathon, but with no guarantee of crossing the finish line. Despite the hefty investment of time, effort and resources, the success rate for bringing new drugs to market hasn’t improved in recent decades. The American Council on Science and Health estimates an average success rate from 2000 to 2015 of only 13.8%, with costs reaching hundreds of millions per drug. The situation appears more grim with the average cost of developing a drug surpassing $2 billion, according to Deloitte.
“Despite increased understanding of diseases and technologies for drug discovery, developing new medicines remains an unpredictable endeavor filled with many failures,” said David Dornstreich, chief commercial officer and general manager U.S. at QuantHealth.
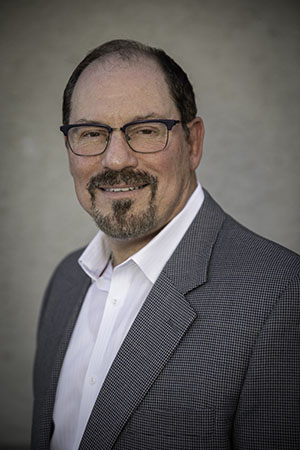
David Dornstreich
It’s no wonder then, given the new-found levels of interest in artificial intelligence that drug developers would attempt to predict the efficacy of an experimental drug at the clinical trial phase. QuantHealth handles more than one trillion data points from the clinical and pharmacological domains. These data points cover more than 350 million patients, more than 100,000 distinct molecules, and more than 180,000 clinical trials.
“Let’s face the facts — 90% of drugs that make it to clinical trials don’t make it to market,” said Orr Inbar, co-founder and CEO at QuantHealth. “They fail somewhere between phase 1 and phase 3.”
The prospect of AI clinical trial simulation still raises eyebrows
A number of companies are exploring the use of AI to help tame clinical trial complexity. Using technologies like machine learning to predict clinical trial outcomes remains rarer. Despite the tangible excitement surrounding the potential of AI in clinical trials, a note of caution still prevails. As futuristic and groundbreaking as these developments may sound, they still verge on the realm of science fiction, and that can naturally lead to a degree of skepticism. QuantHealth’s conversations with pharma companies reveals “mostly excitement,” Inbar said but also some skepticism. “Let’s be honest, what we’re doing is science fiction in the medical realm,” Inbar said. While QuantHealth’s goal is to predict how patients will respond to experimental drugs, the “onus is on us to prove that we can actually do that,” Inbar said.
In October, QuantHealth secured $2.6 million in seed funding to advance its clinical trial simulation platform. This platform enables pharmaceutical companies to virtually test thousands of trial protocols, evaluating design variations to pinpoint the optimal approach.
The concept of using machine learning to predict clinical outcomes is not entirely new. In 2019, researchers at MIT employed AI and statistical techniques to enhance data on clinical trial outcomes, aiming to provide more timely and accurate estimates of the risks involved.
In addition, an MDPI study reported that machine learning models could predict outcomes of prostate cancer clinical trials using bicalutamide, based on data from three phase 3 trials. The best models achieved 76% accuracy.
Learning from other industries
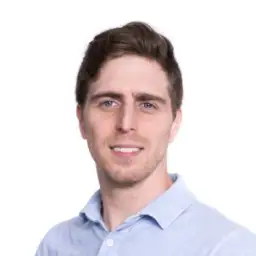
Orr Inbar
Inbar used the analogy of designing rockets or semiconductor CPU chips to illustrate how different industries are leveraging technology to solve major challenges. He said, “Before these items go to production, they go through endless and rigorous computer simulations to figure out the best way to build them. Yet, in drug development, we’re still following the same processes we’ve used for literally over 100 years. The only way to test is on real patients, even though the stakes are so much higher.”
Inbar pointed out the long waiting periods and astronomical costs associated with clinical trials, even when the odds of success are grim. “It’s mind-boggling,” he said. But Inbar is optimistic that technology and data can turn the tables. He argued that we could flip the model on its head with AI clinical trial simulation, automating drug discovery processes before taking them into clinical stages. “Can we take all this technology and data that we have at our disposal and flip this model on its head?” he asked.
Inbar underscored the potential of pivoting to in silico methodologies. The idea is to rely heavily on automation and simulation prior to embarking on clinical production, which could result in substantial risk mitigation and optimization of the entire process. “We’ll never be perfect,” he conceded. “We’ll probably still need to test drugs on patients, but you’ll need much fewer patients and you’ll do it much less often. It’ll require a fraction of the current time and cost if we approach this intelligently and more efficiently.”