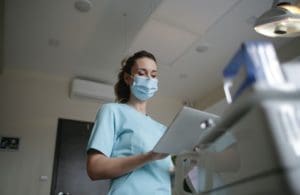
Photo by Andrea Piacquadio from Pexels
Cardiology trials often adopt composite endpoints that combine several events of clinical interest as the primary efficacy outcome.
Time-to-first-event approaches follow the recommendations of regulatory agencies. But composite outcomes that only consider the first event are suboptimal for a chronic disease such as heart failure (HF), which is characterized by recurrent HF hospitalizations since repeat events within individuals are ignored in analyses.
Recurrent HF hospitalizations indicate a worsening condition and disease progression, so considering all HF hospitalizations in analysis more accurately assesses the effect of treatment on the true disease burden. Currently, there is no recommendation as to the preferred approach. The CHARM-Preserved trial shows the impact of analyzing only the time-to-first event and ignoring repeat hospitalizations.1
Of the 508 patients hospitalized at least once for heart failure (HF), 209 of these patients were hospitalized a second or more times with HF thereafter. In total, 939 HF hospitalizations occurred, which means that a time-to-first-event analysis threw away 431 hospitalizations. Even though there is controversy as to which statistical methods are most appropriate, quantifying the effect of treatment on non-fatal recurrent events remains vital.2
Time-to-first-event analysis of composite endpoints is the gold standard in statistical approaches and is supported by its substantial experience and use with regulatory assessment. While there’s less regulatory experience for recurrent event endpoints and complex statistical approaches, it’s important to consider these methodologies because of their many advantages.
Methods for analyzing recurrent events
Methods for analyzing recurrent events can be divided into two categories: time-to-event approaches and methods based on event rates. Time-to-event methodologies include the Wei-Lin-Weissfeld (WLW), the Prentice-Williams-Peterson (PWP) and the Andersen-Gill. Methods based on event rates are the Poisson and the negative binomial.
The WLW model examines the cumulative time from randomization to K ordered events. It considers each event in turn as the outcome in a sequence of applications of the Cox proportional-hazards model.3 Its distinctive feature is that each individual’s time at risk for events (i.e., first, second, third event etc.) begins at randomization. This way, full randomized treatment groups are compared.
The WLW model preserves randomization and is semi-parametric, so there is no assumption on the baseline hazard. However, issues surrounding the interpretation of the estimated hazard ratios can be a disadvantage as the treatment effect for a second hospitalization is cumulative, considering the effect on the first. If a large treatment effect is observed for the first hospitalization, this will impact the treatment effect for subsequent events. Global effects can also be difficult to interpret if the estimated hazard ratios are combined. Furthermore, analysis must be restricted to a K subset of all hospitalizations due to fewer higher-order events, so this methodology does not analyze all hospitalizations. With the CHARM-Preserved data, for example, it is sensible only to consider the first three or four hospitalizations for analysis, so patients with five or more hospitalizations will still have some of their events ignored.
The PWP model is similar to the WLW, but gap times (i.e., the times between consecutive events) are considered in analysis resulting in conditional risk sets.4 Analysis continues in the same manner, however. Distinct hazard ratios are estimated with associated p-values for K gap times. The PWP model presents with many of the same advantages and disadvantages as the WLW model. The main difference is that conditional risk sets in the PWP model better reflect the true disease progression but do not maintain randomization like the WLW model.
The Andersen-Gill
The Andersen-Gill is an extension of the Cox proportional-hazards model that analyzes gap times.5 In the Cox proportional-hazards model, each individual’s time-to-event contributes independently to the partial likelihood. In the Andersen-Gill model, each gap time contributes independently, giving a hazard ratio-like intensity ratio for the treatment effect. Advantages of the Andersen-Gill include the fact that it is a semi-parametric approach and can analyze all individuals’ hospitalizations. A disadvantage is that it assumes a common baseline hazard for all of the gap times, which may not be true in practice.
Poisson and negative-binomial
Methods based on event rates include the Poisson and negative binomial distributions. The Poisson model considers the total number of events divided by the total follow-up in each group, giving a rate ratio for recurrent events. This distribution assumes that the underlying event rate is the same across all subjects (and follows a Poisson process) and assumes independence of events, which is not a sensible assumption in heart failure hospitalizations as hospitalizations within individuals are likely to be related to each other.
The negative binomial distribution naturally induces an association between repeat events within individuals through a random effect term that follows a gamma distribution. The negative-binomial assumes individual-specific Poisson event rates conditional on a random effect for each patient’s true underlying rate. It is easy to implement, does not require complex data files and the resulting estimated rate ratio is easy to interpret and can comfortably be communicated to non-statistical audiences.
In conclusion, the world of recurrent events in HF studies is complex, and there is no obvious right answer. There is still a tremendous amount of work still to be done in this area, and I look forward to seeing how this field of statistics develops in the future.
References
- Yusuf, S., M.A. Pfeffer, K. Swedberg, C.B. Granger, P. Held, J.J.V. McMurray, E.L. Michelson, B. Olofsson, J. Östergren. Effects of candesartan in patients with chronic heart failure and preserved left-ventricular ejection fraction: the CHARM-Preserved Trial. The Lancet. 2003; 362:777-781.
- Rogers, J. K., S. J. Pocock, J. J. McMurray, C. B. Granger, E. L. Michelson, J. Östergren, M. A. Pfeffer, S. D. Solomon, K. Swedberg, and S. Yusuf. Analysing recurrent hospitalizations in heart failure: a review of statistical methodology, with application to CHARM-Preserved. European Journal of Heart Failure. 2014; 16(1), 33–40.
- Wei, L. J., D. Y. Lin, and L. Weissfeld. Regression analysis of multivariate incomplete failure time data by modelling marginal distributions. Journal of the American Statistical Association. 1989; 84(408), 1065–1073.
- Prentice, R. L., B. J. Williams, and A. V. Peterson. On the regression analysis of multivariate failure time data. Biometrika. 1981; 68(2), 373–379.
- Andersen, P. K. and R. D. Gill. Cox’s regression model for counting processes: A large sample study. Annals of Statistics. 1982; 10(4), 1100–1120.
Jennifer Rogers is the head of statistical research and consultancy at Phastar, a contract research organization. Rogers joined the company in 2019, following a move from the University of Oxford, where she was director of statistical consultancy services and an associate professor in the department of statistics. She had previously worked as a post-doctoral research fellow in the Department of Statistics funded by the National Institute of Health Research.