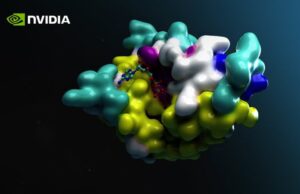
NVIDIA BioNeMo AI molecular modeling software can uncover complex biochemical interactions through AI-driven molecular modeling techniques. [Image courtesy of NVIDIA]
Technically, graphics processing and AI hardware powerhouse Nvidia is also a drug discovery company. It may not discover drugs in-house, but it has developed BioNeMo, a comprehensive generative AI platform for drug discovery and Clara, a collection of healthcare frameworks, applications, and tools, including for biopharma. Nvidia partners include Amgen, AstraZeneca, GSK and Insilico Medicine.
Similarly, biotech pioneer and Roche subsidiary Genentech is also an AI company. It has experience in applying machine learning to an array of disease areas, and has extensive biological and molecular datasets and research capabilities. Its initiatives include alliance with firms such as Recursion Pharmaceuticals and Reverie Labs that focus on using AI for novel target discovery and the development of next-gen therapies.
Now, Nvidia and Genentech have forged a multi-year alliance to both accelerate the process of drug discovery and development while also expanding the scope of computational and generative AI research. With a shared vision regarding the promise of AI in drug discovery, Nvidia and Genentech are combining their strengths to tackle the immense challenge of sifting through the vast space of potential therapeutic molecules and proteins. Genentech anticipates that the partnership will bolster its AI research programs by advancing its generative AI models and algorithms into what it calls “a next-generation AI platform” to streamline drug discovery.
Analysts, too, are generally optimistic about the potential of AI as a transformative force in the pharmaceutical industry. According to McKinsey, for instance, the convergence of computational power and biological research could lead to unprecedented gains in the pace of drug discovery. Similarly, Boston Consulting Group agrees that AI could significantly alter the economics of innovation in drug discovery, possibly allowing new medicines to be discovered for a broader range of conditions and by a more diverse research community. Conversely, UBS has a more measured take, seeing generative AI in particular as more evolutionary than revolutionary.
Yet adoption remains at an early stage, and the early phases of the clinical journey of AI-designed drugs have seen mixed results to date. For instance, some AI-generated compounds, such as Exscientia’s EXS-21546, initially showed promise but faced challenges later on, leading to the termination of its phase 1/2 trial. BenevolentAI’s BEN2293, in a phase 2a study, was found safe and well-tolerated, but did not reach statistical significance for mild-to-moderate atopic dermatitis secondary endpoints. Meanwhile, Insilico’s INS018_055 is now advancing into a Phase 2 trial to test its potential as a first-in-class anti-fibrotic small molecule inhibitor.
Deciphering the boundless frontier of drug discovery
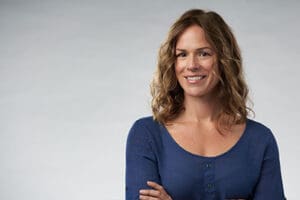
Kimberly Powell
To illustrate the enormity of the universe of possible molecular structures, vice president of Healthcare at NVIDIA, Kimberly Powell, referenced the number of compounds in the chemical space, which is estimated at 1060. For reference, some estimates peg the number of atoms in the universe at around 1080. “The number of potential proteins is even more vast at 10160,” she said in a recent media briefing.
By contrast, a typical screening approach might consider between 5,000 to 10,000 compounds for each new drug. While some research groups have succeeded in screening upwards of 11 billion compounds, that’s still a metaphorical drop in the bucket compared to the entire universe of chemicals and proteins, which Powell described as “a near infinite search space.”
NVIDIA created BioNeMo, a comprehensive generative AI platform for drug discovery to help tame that complexity. “What other than generative AI would allow us to search this enormous complex space?” she asked. “We can search it for targets, we can search it for leads, and we can even intelligently search it for optimized molecules,” she said.
When one thinks about identifying a target, there are thousands of genes, millions of variants, and trillions of cells that make up the human body, agreed Dr. John Marioni, senior vice president and head of computational sciences at Genentech Research and Early Development (gRED). “Identifying which one of these different processes to target is itself hard enough, but then, you add the complexity of inter-individual variability onto this, along with the fact that there are tens of thousands of diseases, and you can imagine that target identification is really challenging,” Marioni said. “And once you’ve got that target, discovering an antibody to target it, or discovering a small molecule that’s going to have the effect you want, is again, a challenge in a trillion-trillion parameter space.”
Technology meets biology: Inside NVIDIA and Genentech’s strategic alliance
The alliance between Genentech and NVIDIA will use NVIDIA’s DGX Cloud to bolster Genentech’s machine-learning algorithms and models. DGX Cloud is a scalable platform for AI and deep learning applications, suited for a range of applications across industries.

John Marioni
In the Genentech-NVIDIA partnership, DGX Cloud will provide dedicated instances of AI supercomputing and software to accelerate drug discovery models. In general, Genentech anticipates the collaboration with NVIDIA will vastly accelerate how quickly it can train and use its foundational models. “And most importantly, this is going to change how we discover and develop medicines that impact lives across the world,” Marioni said.
BioNeMo, NVIDIA’s specialized platform for generative AI in drug discovery, will help Genentech customize and scale up its drug discovery models, allowing Genentech researchers to pretrain or fine-tune models on DGX Cloud and integrate them into their computational drug discovery workflows.
The collaboration will initially focus on optimizing Genentech’s AI models within its “lab in a loop” framework, which uses experimental data to inform computational models and improve molecular designs. This iterative framework uses experimental data to inform and optimize future molecular designs through generative computational models.
“This lab in a loop requires multiple things to make it work, the ability to generate and the possession of massive volumes of data,” Marioni said. It unifies Genentech’s access to hundreds of petabytes of proprietary data of numerous types. “And you could complement that with public data,” Marioni added. The concept also unifies expertise in the biological and the clinical sciences as well as in AI and computation.
And to that latter point, Genentech recently established Genentech Research and Early Development (gRED) Computational Sciences with more than 400 employees focused on applying advanced computational methods and machine learning to drug discovery and development. “It is an intrinsic and core part of the Genentech Research and Early Development portfolio,” Marioni said.
Under the terms of the deal, Genentech will retain control over its proprietary data in the partnership unless it decides to share such data in a given project in the collaboration.
Potentially expanding the horizons of the NVIDIA-Genentech AI pact
While the initial focus of the collaboration is on early target discovery and the development of molecules, it is possible that it could expand over time. “From an operational perspective, [AI has] had huge impacts in other industries in the manufacturing space,” Marioni said. “It could be a very interesting opportunity to explore that as we move forward.”
NVIDIA’s BioNeMo provides a foundational model that includes an index of desirable properties for molecules. “By injecting these properties into the generative and predictive capabilities of the model, we can effectively guide its development,” Powell said. “What may not be immediately successful in manufacturing can be preemptively addressed by incorporating these properties upstream, she added.
The BioNeMo platform’s capabilities include biomolecular models for 3D protein structure prediction, de novo protein and small molecule generation, property predictions, and molecular docking simulations. “These models [within BioNeMo] have become so powerful. They can essentially use multi-property optimization, just like diffusion models.” In general, diffusion models, a versatile type of generative model in machine learning, support an array of applications from upscaling images to creating realistic simulations. In drug discovery, they can generate complex molecular structures for drug design, and explore how molecules move within the body.
The sophistication of current research models allows them to address multi-optimization problems. “I think you’re going to be able to see a nice complement, with these models going back and forth across that chain, enhancing each stage of the research process,” Powell concluded.