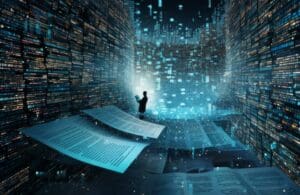
[Kishore Newton/Adobe Stock]
In drug discovery and development, data sources are as diverse as they are plentiful. There are comprehensive databases brimming with molecular targets, cellular processes, genomic sequences, proteomic profiles, and metabolite patterns that shed light on disease pathways. Data possibilities in the patient care realm are similarly vast, spanning electronic medical records, imaging datasets, and even patient-reported outcomes and adverse events reported on social media. The biomedical research site PubMed has tens of millions of research articles and studies.
Yet, it’s easier to drown in such turbulent data volumes than it is to swim. Various estimates over the past decade have projected that 80% of healthcare data are unstructured. “There’s a huge amount of information that’s not standardized,” said Jane Reed, director of life sciences with Linguamatics, an IQVIA company.
Harnessing NLP in drug development
Enter natural language processing (NLP). A subset of AI focused on enabling computers to understand and process human language, NLP opens up new vistas of biomedical information that comes from unstructured data. Although capable on its own, the real potential of NLP comes from pairing it with structured data sources, powering approaches like supervised learning, deep learning and random forest models. “You can combine your unstructured data with your structured data that you already manage with all these algorithms, then you’ve really got the best substrate for your decision support,” Reed said.
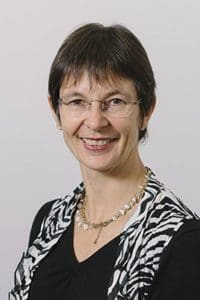
Jane Z. Reed
Of course, the ultimate aim of using NLP — or any other AI technique — is to ensure sound safety and efficacy profiles of potential drug candidates.
The practicalities and challenges of real-world drug deployment
But despite advances in computing horsepower, data availability and AI algorithms, the industry still grapples with a high failure rate. “Humans are hugely complex,” Reed noted. The variability, even in controlled clinical trials, can be staggering. And once a drug enters the real world, the challenges multiply. “When you think about that drug in the real world, you might suddenly be giving that drug to hundreds of thousands of individuals,” she added.
The flipside to medication used in real settings is the fact that drug developers have access to additional tools to spot and understand adverse events. For instance, patients might discuss adverse effects or unexpected benefits on online platforms. On social media, for instance, someone might share, ‘’’I took this drug, and my foot swelled up,’” Reed noted.
In terms of clinical post-market safety, it’s critical that any adverse events in trial patients are recognized as soon as possible. “You want to know now,” Reed said. “You want to know what happened yesterday, what happened in the last week.”
Added to that is the wealth of information regularly published online such as papers, abstracts, and manuscripts. Regulatory agencies can also offer a treasure trove of insights. “Anytime a drug goes through approval, a pharma company will submit a lorry load of documents, and the regulators will review those and create summaries.”
“If you’re running a study, with a particular drug, you may want to know,“ “Has this reaction or event been encountered with this kind of drug before? Has it been seen with the drug target that I’m investigating before? Has it been seen in humans or other species? What’s the mechanism? How do I understand what I’m seeing in my project, from the external data?” she added, highlighting the intricacies of the process.
NLP in drug development can help create a collective knowledge base
NLP, paired with an array of data sources, can help establish a sort of collective knowledge base.
Techniques like NLP can not only help drug developers sift through the massive amounts of data, but also to pinpoint the nuances, patterns, and insights that might otherwise go missed. In the longer term, drug developers could get better at spotting the “right” elements in their research. Clearly, that quest is not new. The emphasis on using data to identify the optimal factors in drug development has a longstanding focus of the industry.
For instance, in 2014, Nature Reviews published a seminal review of AstraZeneca’s small-molecule drug projects from 2005 to 2010. The analysis led to the development of the ‘5R’ framework which prioritized the selection of the “right” elements across the board: the right target, the right patient, the right tissue, the right safety profile, and the right commercial potential.
AstraZeneca’s subsequent adoption of the framework marked a transition toward a more critical approach of drug candidates. The ripple effects of such frameworks led to the industry to ramp up its stringency in reviewing early-phase drug candidates. “It became much easier for kind people to say, ‘Look, this drug should fail, and it should fail now,’” Reed said. “We’ve got this whole mantra of failing early.”
The evolving role of AI in pharmaceutical research
One of the ways that tools like NLP — as part of a comprehensive data-driven strategy — could help is by helping drug developers get better at spotting the “right” elements. Drug developers, by integrating unstructured and structured data sources — both internal and external — can paint a more complete and nuanced picture of potential drug candidates. This holistic view, in turn, can facilitate more informed decision-making, anticipate potential hurdles, and capitalize on opportunities.
While the promise of AI predicting, say, the safety and efficacy profile for a given candidate remains a lofty goal, its immediate power is growing in terms of devising “better models to predict toxicity and safety issues,” Reed said. “That’s growing by the day.”