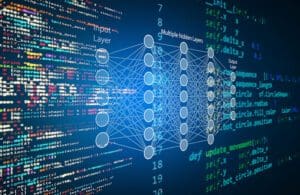
[pdusit/Adobe Stock]
For all of the talk about AI in drug discovery and development, few Big Pharmas are putting up big bucks in AI spending. A CRB survey from late 2023 painted a conservative picture: about half of drug developers planned on allocating between $1 and $10 million for data and AI projects over the next two years. A mere 3% reported budgeting more than $50 million. No companies surveyed had budgeted more than $100 million.
By contrast, Microsoft has invested more than $10 billion into OpenAI alone. Amazon invested $4 billion into Anthropic for minority ownership and a board seat, while Google invested $2 billion plus potentially hundreds of millions more into Anthropic and Character AI for AI research and development. In early 2023, IDC projected that cumulative AI spending would reach $154 billion by the end of the year — 27% higher than a year earlier.
While a growing number of pharma and biotech companies plaster “AI” on their website, few have mature AI strategies that are integrated across the organization. “The first question I ask is, ‘how many people are actually working on AI,’” said Martin Brenner, CEO and chief scientific officer at iBio, an AI-focused biotech. “If it’s one or two, you’re not an AI company. NVIDIA is an AI company. OpenAI is an AI company.”
And while tech companies have benefited from exponentially powerful technologies, thanks to Moore’s Law, which predicted that the number of transistors on a microchip would double approximately every two years, the pharma sector has not seen such benefits. The term known as ‘Eroom’s Law,’ a sort of inverse of Moore’s Law, observes that the cost of developing new drugs has been doubling approximately every nine years since about 1980.
Big Pharma stepping up its AI game
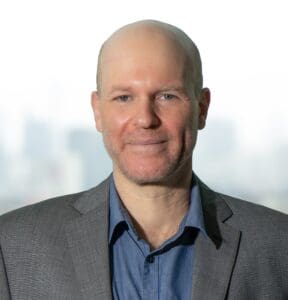
Martin Brenner, CEO and chief scientific officer at iBio
Part of the AI’s promise is to drive efficiency, and now, the pharma industry’s AI ambitions, however, are growing more ambitious. Recent landmark deals in the industry point to a more aggressive push towards integrating AI into the drug discovery process. Cases in point include Nvidia alliances with Genentech and Amgen. A Boehringer Ingelheim pact with IBM. And particularly Eli Lilly and Novartis partnerships with Isomorphic Labs, a subsidiary of Alphabet.
“Increasingly, the kinds of partnerships I think will characterize 2024 and that will be most meaningful in our space are not ones between, necessarily, pharma companies and AI drug discovery companies,” said Jen Nwankwo, Ph.D. While those alliances are important, the partnerships that “will move this space in a new way are the partnerships between AI drug discovery companies and Big Tech,” Nwankwo added.
Potentially bigger Big Pharma AI deals

Jen Nwankwo, CEO of 1910 Genetics
The financial terms of the Lilly and Novartis deals point to stronger enthusiasm in the potential of machine learning, deep learning, reinforcement learning, and generative models in drug discovery. The Lilly deal, a multi-year strategic research alliance to discover small molecule therapeutics against multiple undisclosed targets, includes the potential for up to $1.7 billion in milestone payments plus royalties. The Novartis collaboration is similarly striking. Focusing on the discovery of small molecule therapeutics against three undisclosed targets, the deal could include up to $1.2 billion in milestone payments in addition to royalties.
While many drug developers have clear aspirations to become increasingly AI savvy in the coming years, the fundamental incentives and metrics for success in pharma limit how pervasively these technologies can permeate established Big Pharma players. AI and computational drug discovery may accelerate parts of the R&D process, but Big Pharma companies often have little incentive to overhaul infrastructures that have been established and optimized over decades. While speculative tech moonshots have their allure, a conservative ethos persists in the high-stakes, clinically-grounded, highly-regulated realm of pharma.
Drug developers need ‘vertical AI’
That’s not to say that big change isn’t coming eventually. Technologies like OpenAI’s ChatGPT have signaled the power of AI algorithms to streamline administrative tasks and some regulatory processes when paired with human oversight. But such platforms aren’t likely to spark wholesale change in the industry in the near term. Platforms like ChatGPT, Anthropic’s Claude, and Google’s Bard are an example of what Nwankwo calls “horizontal AI.”
Drug developers ultimately need “vertical AI,” Nwankwo said. “I think each industry has to figure out what the next 10 to 20 years mean for them in terms of their journey with generative AI,” Nwankwo said. Mature vertical AI could be especially powerful in pharma, given its potential to make sense of disjointed, fractured data sets and replace legacy infrastructures. Technologies such as graph neural networks, natural language processing, self-supervised learning models and quantum computing show particular promise on this front over the long term.
But pharma also needs new AI tools, Nwankwo said. “We need new types of models and machine learning architectures that haven’t been built yet, which are beyond the expertise of a biotech company,” she explained. “That’s where big tech companies come in.”
Differing incentives
Big the tech industry, of course, isn’t focused on acquiring rights to specific drugs or therapeutic assets. They are focused on continually improving underlying infrastructure that they can offer as business offerings across a range of sectors, including narrower tools for specialized applications, including in fields like healthcare, biotechnology, and pharmaceuticals.
Big Tech companies can partner with drug developers to build new infrastructure — a sort of rising tide to lift all boats. Consider the impact of standardization in various aspects of AI in drug discovery,” Nwankwo said. This includes standardized datasets, model training, and computing optimization. Standardization is crucial across data creation, exploitation, curation, cleaning, and storage. “It also applies to models in terms of inference, training, and identifying ideal architectures for different therapeutic modalities like cell therapy, gene therapy, antibodies, and small molecules,” she added.
It may sound obvious, but Big Tech companies are best positioned to develop the hardware and software to develop cutting-edge machine learning algorithms, models, and scalable infrastructures. Big Tech companies can work with drug developers to create standardized computing infrastructure that supports scale. “What they [Big Tech firms] don’t have are the [proprietary pharma and biotech] data sets,’” noted Martin Brenner, CEO and chief scientific officer at iBio.