Lantern Pharma’s AI-powered sprint

[Adobe Stock]
Lantern Pharma (NASDAQ: LTRN), a publicly traded clinical-stage biotech company with a market cap of around $79 million as of mid-March 2024, is shooting for developing $200 million drugs with a machine learning-based platform.
The oncology-focused firm Lantern Pharma, profiled last year, has developed a new drug (LP-284) in less than three years for under $3 million, which CEO Panna Sharma notes is “unheard of.” By using AI, Sharma projects that the company could develop a drug from concept to phase 3 trials for a price tag of $100–200 million — a small fraction of the typically $2.3 billion drug development price tag.
“We’re developing new drugs in less than two and a half years, from an idea through GMP manufacturing, to orphan indications, and multiple publications at ASH [American Society of Hematology] and SOHO [Society of Hematologic Oncology],” he said.
In all, the company has three drug candidates in clinical trials: LP-184 (phase 1 for solid tumors), LP-284 (phase 1 for lymphomas and sarcomas), and another in phase 2.
Rethinking drug development cost estimates
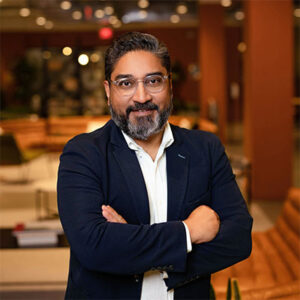
Panna Sharma
Sharma argues that widely accepted drug development cost estimates need careful interpretation. While they have their place, he emphasizes that these figures often represent “aggregate spending” across clinical trials, including failures and post-approval (phase 4) programs. Sharma contends that the actual cost for a successful drug to reach approval is closer to $800–900 million, particularly for small to mid-sized biopharma companies.
To arrive at that calculation, Sharma notes that he worked with academics and also internally, “When you skew it more towards small-mid sized biopharma, that number gets to that and sometimes lower.
Unravelling AI’s promise to slashing drug development costs by millions
Using AI, “you should be able to progress from a concept through a phase three clinical trial for less than $200 million.” With Lantern’s approach, “we could probably even do it for closer to $100 million,” Sharma said.
Core to Lantern’s strategy is amassing data. Its RADR (Response Algorithm for Drug Positioning & Rescue) AI platform recently surpassed 60 billion data points. “In the last year, we’ve scaled RADR to a whole different level,” Sharma noted.
The RADR dataset includes a plethora of sources, including drug sensitivity data, combination treatment outcomes, biomarker data in rare cancers. Also in the mix are data on emerging synthetic lethal targets, genome and methylome datasets across different cancer types, and immuno-oncology related data like antigen, immune-response, and protein data.
Lantern sources the data from a mix of sources, including proprietary sources, collaborations, and public databases. The company aims to reach 100 billion data points by the end of 2024. The platform incorporates large-scale biomarker and drug-tumor interaction data to provide insights into how drugs interact with tumors and predict potential responses across cancer types and subtypes.
Building a data foundation
Despite the growth in data points, Lantern has been able to maintain a lean team of fewer than 30 employees. Advances in Lantern’s AI capabilities have driven efficiency, allowing it to generate and evaluate millions of algorithms quickly. “Eventually, the algorithms have to know how they’re performing against the questions that you ask, which they do,” Sharma said “And the algorithms can start teaching themselves. There are meta-learner tools now that are available. These meta-learner algorithms are very good if they’re focused on specific problems. And so that’s what we’ve deployed now in a pretty advanced DevOps environment called ML Flow,” Sharma explained.
Despite the growth in data points, Lantern has been able to maintain a lean team of fewer than 30 employees. Advances in Lantern’s AI capabilities have driven efficiency, allowing it to generate and evaluate millions of algorithms quickly. Sharma explains the power of this approach: Eventually, the algorithms start to evaluate their own performance against the questions researchers ask. “The algorithms can start teaching themselves,” Sharma said. Lantern uses the power of meta-learning tools to amplify its research. “These meta-learner algorithms are very good if they’re focused on specific problems. And so that’s what we’ve deployed now in a pretty advanced DevOps environment called ML Flow,” Sharma explained.
The emerging trend of AI in drug discovery
Lantern is part of a growing wave of biotechs using AI to transform drug discovery. Pfizer used cloud-based supercomputing with AI machine learning models to develop Paxlovid, its COVID antiviral, screening millions of compounds and cutting computational time by 80-90%, ultimately designing the drug in four months. Amgen is aiming to shave about two years off the development times by 2030 through AI-based clinical trial optimization. Similarly, Iambic Therapeutics recently announced that its AI-discovered drug candidate IAM1363, a selective HER2 inhibitor, moved from program start to clinical trials in under two years.
That doesn’t mean implementing AI technologies at scale is straightforward in a highly-regulated industry. Incumbent pharma companies tend to face cultural and organizational barriers to fully exploit emerging technologies. “The biggest challenge in the pharma industry isn’t the adoption of technology. It’s the generational change that needs to occur,” Sharma said. “And other industries like tech, for example, or even finance or retail, that generational change has occurred a lot faster and swifter than in pharma,” Sharma noted.
More shots on goal
While drug development, even with AI, can still be a gamble, Lantern’s approach could enable the company to take more “shots on goal” by significantly cutting the time and cost required to bring a drug from concept to clinical trials. “Data gives us this advantage because we enter the lab with greater clarity, having a meaningful idea of patient response associated with a biologically feasible signature much earlier,” Sharma said. “We know which patients are likely to respond versus those that may or may not, earlier.”
While Lantern’s technology shows promise, the path from petabytes to cancer patients is long and not without risk. “I can’t guarantee it will always work,” Sharma said, but the economics of investing a few million in a promising candidate could be transformative. “If I could invest $3 million and generate a novel idea that’s human-applicable, I would do it 20 times a year. Even if only five are successful, that would be fantastic.”