Amgen (Nasdaq:AMGN) is sharpening its focus on generative biology, an emerging discipline that can accelerate protein drug discovery.
The company describes generative biology as “a revolutionary approach to drug discovery and development” that enlists the help of AI and machine learning to design novel protein therapeutics.
Although the term generative biology may be new, Amgen has invested in the marriage of wet lab high throughput automation and dry lab computational biology for decades.
A recently created internal team known as the Digital Biologics Discovery Group aims to expand its focus on generative biology. Leading that group is Alan Russell, who is currently Amgen’s vice president of biologics. Before joining the company, Russell founded and led the McGowan Institute for Regenerative Medicine at the University of Pittsburgh. He also created and led the Disruptive Health Technology Institute at Carnegie Mellon University.
In the following interview, Russell shares his vision for the nascent field of generative biology, touches on the promise of quantum computing and provides an update on the recent acquisition of Teneobio and partnership with Generate Biomedicines.
The responses have been lightly edited.
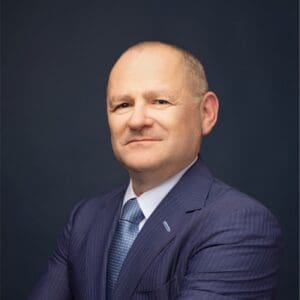
Alan Russell
Drug Discovery & Development (DDD): Could you provide an overview of your background?
Russell: I started out as an enzyme engineer by background. At the beginning of my independent research career, I was very interested in engineering enzymes and addressing core weaknesses of enzymes by either changing the enzyme themselves or adding polymers to enzymes.
I’ve been somewhat involved in drug development as well. I’ve looked at protein-based therapeutics and have done a lot of work in regenerative medicine, biomaterials and cell therapy. So I’ve been lucky enough to have a fairly broad scientific background.
If you think about what you’re trying to accomplish with regenerative medicine, you’re trying to use molecules or cells to intervene in a conversation inside the body and to influence cells and tissues to do things that you might not normally choose to do. So I think it was quite natural that my interest in engineering proteins led to an interest in how those proteins could be incorporated into materials or expressed from cells in such a way that typically, we’re interested in how to swap inflammation for regeneration.
DDD: What inspired you to join Amgen in 2020?
Russell: Amgen has a long and storied history of discovering biologic and protein-based drugs. That goes back all the way actually to when I was in graduate school. Then, Amgen was forging the path forward of inventing what biotechnology companies did for human health. That world-class team is extraordinary.
I need to differentiate between my personal work and the work of the hundreds of scientists at Amgen. This is a team-based sport. I help the team in any way I can, but the work is theirs.
For a very long time, I think Amgen has believed that the timeline of biologic discovery takes too long. This timeline involves figuring out the right protein to make for a therapeutic impact and then how to perfect it and turn it into a drug. It takes years, and it doesn’t always succeed.
When you think of the waves of new science that can transform discovery timelines and discovery success rates, the questions become, “What are some of these key areas where science is changing? And how can we draw that into drug discovery to enable the transformation of drug discovery?”
There is computer science, digital biologics and discovery as key elements. And certainly, if you think of what’s happening with artificial intelligence, machine learning, and all the different models that have been developed, it’s not that computer scientists took a set of tools. They’re now deploying that set of tools across lots of different industries. They’re inventing new computer science all the time.
At Amgen, we want to harness years and decades of investment in automation and data generation. We were skating to where the puck was going. For the last 10 years, the company has strategically invested in being ready for when AI, machine learning and other computational techniques can truly help us. I think what’s exciting is we’re at that time right now.
DDD: How does all of this relate to generative biology, and how can it help drug developers understand the links between sequence, structure and function?
Russell: The nice thing about generative biology is that it doesn’t have a meaning everyone would necessarily agree to. It’s like the phrase ‘regenerative medicine’ 20 years ago. When we started using that word, the first question people asked was, ‘Well, what is regenerative medicine?’ People defined it differently, but eventually, it settled down.
Generative biology is similar in that way. It’s a word that’s only been added to our scientific lexicon within the last couple of years, which means we are inventing it. But the core elements of how you do generative biology and how it impacts drug discovery have been in place for decades.
Generative biology entails the idea of extracting generalized principles that define how biological systems function. By doing that, you bring together life science, computer science, ultra-miniaturization, automation and robotics. That involves the seamless fusion of the wet lab with the dry lab.
Current life science and automation platforms are really advanced. I mean, We’re very good at generating large datasets. And the more we learn about what AI, and machine learning can do in the drug discovery process, the more we learn how to fine-tune data extraction to reach the end goal. And that end goal is to unveil this link between sequence, structure and function.
Some of my colleagues in the industry, I think, look at generative biology as maybe something that’s a little too new — a little unproven. I really don’t. If you look at biologic drug discovery, Amgen has been pioneering that for 40 years, along with an entire industry. It’s highly sophisticated. The same is true in robotics and automation and the wet lab side of large-scale data generation. It’s all highly advanced. There are decades of sophistication. And the entire tech industry is a long way down the line of sophistication with AI, machine learning and generative models — not applied to drug discovery but just applied broadly.
The real trick is how do you take these three major areas and bring them together in an interdisciplinary way so that you take three advanced areas of science and then merge them to do generative biology?
DDD: The promise to integrate biologic drug discovery, robotics, automation and AI sounds exciting.
Russell: For me, it’s phenomenally exciting, and it is very much like pressing the repeat button. I remember in the early days of regenerative medicine, there was this thought that you could go from organ transplant — treating patients with an entire organ — to treating patients with cells. That was like a huge, exciting leap of faith. It is similar to this idea of generative biology. We can do things at a pace and success rate that was never achievable before.
The industry is well aware that it takes years to find protein-based lead molecules and then additional years to turn them into drugs. Unfortunately, oftentimes, when you are far down the line, it turns out they didn’t work as predicted. That is a grand challenge. How do you considerably reduce those timelines and increase those success rates?
Amgen is blessed because we have these 40 years of history upon which we can learn and rely on. But we know as well as anyone in the industry how long it takes and how difficult it is to do.
But the process is also really measurable. That’s the beauty of trying new science. And we already know we have the right sets of tools to drive transformation.
DDD: Could you share more about the role you see in software and hardware fueling generative biology advances?
Russell: I’m not sure I would say that quantum computing is inspiring change, but it certainly will impact the change.
Everything that’s been done so far pretty much has been done with traditional hardware and traditional software.
We are deeply interested in this remarkable new wave of quantum computing and quantum-inspired algorithms. We’ve carefully thought about quantum-inspired artificial intelligence and quantum hardware, and how the life sciences can prepare for them.
A large part of the discovery organization at Amgen is to prevent technological surprise. We don’t want to be surprised by new ways of science. Instead, we want to surf those waves when they are cresting and invent new science along the way.
When it comes to quantum computing, quantum-inspired AI, we spent a great deal of time thinking about that, trying to figure out when’s the right time to partner in that space and to identify what life science problems are particularly waiting for new hardware/software solutions. We have identified some challenges that can’t be solved in traditional ways. I’m super excited about that science, and I believe the wave has crested. I think quantum computing will influence our industry. We certainly want to be at the leading edge of that.
DDD: Last year, Amgen acquired Teneobio for $900 million. The company was developing novel biologics known as Human Heavy-Chain Antibodies (UniAb). How does that acquisition affect your group?
Russell: The group is excited. One of the pesky things about trying to make complex proteins and using AI and machine learning is the question, ‘What’s the match between the kind of data we can generate and its ability to be mined with artificial intelligence?’ And the more complex the kind of protein we’re trying to make, the harder it is to draw out the sequence-to-structure-to-function relationships that will fuel the generative biology revolution.
If one’s interested in what you might call ‘sequence-based discovery,’ the idea could be to apply your knowledge to sequences in order to predict their structure and, much later, to be able to think about function. Accomplishing that is much easier with the platform Teneobio developed than with almost any other platform. We feel like our passion and commitment for digital biologics discovery drove that acquisition.
Digital biologics and discovery needs can be partnered seamlessly with the dry lab. So if you have the wet lab and the dry lab, and you haven’t thought about how to design each for the other, you can miss out on that marriage. And we want that marriage to be as good as it can be. So the Teneobio platform, which is now Amgen’s UniAb platform and the UniRat platform that generates those antibodies, is the perfect starting place for data generation and then eventually for sequence-based discovery.
A digital biologics discovery group is seamlessly married to this antibody discovery work.
DDD: In January, Amgen announced a collaboration with Generate Biomedicines focused on a multi-target, multi-modality research collaboration agreement. Can you share more about that?
Russell: If you think about it, our industry must find interesting proteins and turn them into valuable functioning drugs. Those choices along that pathway of discovery are complex. And the more you’ve done it, the more grounded you are about what it takes, and the more you might understand how to create that seamless marriage I mentioned earlier.
We understand where the value lies and where to apply which partnership in this space. We know which drugs might benefit the most from generative biology.
We have a generative biology team, a digital biologics discovery team and a generative biology team. But we’re always looking to the outside, and our door is always open to partnership and collaboration. We don’t own 100% of the good ideas of how to deploy generative biology and couple it with digital biologics discovery. So we felt that Generate was a great fit. There will be others for sure.
And eventually, working together, we will be able to weave together those sequence-to-structure-to-function relationships with generalized principles that don’t exist today.
If you think about the James Webb Space Telescope that is sending back these extraordinary images, we’re able to see those because the scientists and engineers have equations that govern everything about how to understand design, build and set off a trajectory so that we can pinpoint within meters the landing of a rocket that’s traveled millions of miles. That’s extraordinary. There are equations that govern the fundamental principles of how to do all of that. Those equations don’t exist in biology. What we’re trying to do is so much harder because those principles don’t exist.
For instance, Alphabet subsidiary DeepMind’s protein structure predictions showed how you can unpeel that sequence-to-structure relationship. If you can do that, you can begin to design much more precise experiments and find drugs that our patients are waiting for much more quickly.