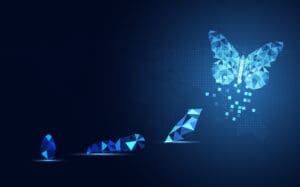
[Adobe Stock]
The value of artificial intelligence (AI) and machine learning (ML) in medical imaging is undeniable: more accurate diagnoses, predictive insights and streamlined workflows. However, as Big Pharma and medical research institutions amplify their AI and ML endeavors, they confront pivotal challenges. Chief among these challenges are the intricacies of labeling and annotating medical images.
Approximately 80% of the time it takes to prepare real-world data for downstream analysis is used on seemingly foundational tasks like locating, curating and structuring data. When we narrow our focus to medical imaging, the stakes rise significantly. The sheer volume of data required to do this work makes using human-only annotation close to impossible. And in this domain, the most minor details can critically influence diagnostic accuracy, making it imperative that data is not only accessible but clearly organized and curated.
The challenges outlined below represent some of the biggest roadblocks in streamlining reader workflows. Real-world data from open data lakes, data brokers and participating trial sites provide countless images of tumor classifications, phenotypic characterizations, drug-targeting biomarkers and cohort identifiers. And that’s just the images. Add in the genomic, proteomic, metabolic and other ’omic’-based data from the clinical side, and the amount of real-world information that needs wrangling to fuel AI and ML modeling is truly mind-boggling.
However, the need to streamline data management is about more than just efficiency. It’s a pivotal factor in ensuring that AI-driven advancements in medical imaging retain the precision and reliability needed to evolve personalized medicine and ultimately influence patient outcomes.=
Challenge 1: Difficulties with reader onboarding, retention and management
The intricacies of medical imaging demand a high degree of specialization. Cross-referencing annotations from three different experts before AI and ML meant hiring in triplicate. That’s neither efficient nor fiscally responsible. Now, some of that work can be outsourced to AI-driven models, but even they need skilled people to maximize output.
When considering the diversity of medical imaging modalities and vast set of skills radiologists need to possess, it’s clear why onboarding and retention pose such significant challenges. Add in long contracts with expensive professionals and the limited number of performance metrics associated with their work, and the inefficiencies in manual image labeling become clear. Although finding talent can be a challenge, ensuring your workforce remains engaged and consistently delivers high-quality results is paramount to success. Mistakes in manual image labeling can compromise the integrity of the data, i.e., the bedrock on which AI and ML models are built.
The solutions proposed below address these specific pain points, ensuring that recruitment, training and managing new readers align with the unique demands of the medical imaging domain. These solutions also create an environment where expert readers can thrive and contribute effectively to the AI-driven research ecosystem.
Potential solutions
- Comprehensive training: Establishing rigorous yet concise training modules to bring readers up to speed on proprietary systems and standards ensures they’re familiar with any new technology.
- Collaborative platforms: Single systems that promote real-time collaboration and feedback between readers and data scientists can build camaraderie and ensure consistency with annotations.
- Specialized onboarding: Given the diverse expertise required in medical imaging, having dedicated onboarding pathways tailored to different specialties can be a huge value-add. It ensures that experts in each field receive the training and resources that are most relevant to the tasks they perform.
Challenge 2: Rigid out-of-the-box workflows and costly customization
In medical imaging, no two projects are identical. With each imaging study or research initiative, there’s a set of unique parameters and needs. Applying a generic, one-size-fits-all solution to such diverse requirements is inherently problematic. The fallout is often a compromised workflow, with bottlenecks and inefficiencies, that can lead to questions in data quality. Additionally, when researchers engage outside engineers to build and tailor systems for specific projects — i.e., create a “home-grown” solution — they encounter costly problems with adoption and scalability. The bottom line is that rigidity and prohibitive customization jeopardize both project timelines and data accuracy.
Championing adaptable workflow engines and seamless integrations establishes a flexible yet structured approach. This approach ensures that each project can be executed efficiently without compromising its unique requirements or draining resources.
Potential solutions
- Adaptable workflow engines: Instead of fumbling around with generic solutions, platforms should offer customizable workflows. Customization caters to the unique requirements of each project without incurring additional costs or delays.
- Integration with commonly used tools: The platform should seamlessly integrate with widely used tools and software common to imaging and AI, ensuring readers and data scientists can use their preferred methods without friction.
- Scalable infrastructure: As projects grow and accumulate more data, the infrastructure should be designed to scale effortlessly. Cloud-based solutions can adjust to increasing workloads, maintaining consistent performance, and preventing bottlenecks.
Challenge 3: Fragmented experiences
The digital landscape of medical imaging is vast, often encompassing tools, platforms and systems designed with specific purposes. But this multiplicity can be a double-edged sword. While there’s specialized software for every conceivable need, home-grown solutions without a unified platform can lead to disjointed experiences. On the surface, it appears to be an issue of convenience, but the implications run deeper.
Fragmentation hampers the productivity of expert readers and data scientists, forcing them to juggle multiple interfaces and calling into question data uniformity and accuracy. When data moves across disparate systems without centralized oversight, the potential for errors, overlaps and misinterpretations grows exponentially. The core challenge lies in the inherent fragmentation of the tools and the subsequent splintered workflows.
Promoting unified platforms, interoperability standards, and integrated feedback loops paves the way for a cohesive work environment. It’s about simplifying tasks and creating an environment where data integrity, efficiency and clarity are at the forefront. This cohesion ensures that the people and the algorithms they feed can operate at peak performance.
Potential solutions
- Unified platforms: A centralized platform that integrates data sourcing, labeling, annotating and quality checks can significantly streamline the workflow and provide a consistent user experience.
- Interoperability standards: Embracing and promoting interoperability standards ensures data can be easily shared, transferred and processed without format or quality issues.
- Feedback loops: Platforms with real-time feedback mechanisms allow readers to instantly communicate discrepancies, ask questions, offer insights and solve problems.
- Automated version control: With multiple experts working simultaneously on a single project, having automated version control systems is essential. It ensures that everyone is working with the most recent and accurate version of the data, eliminating redundancy and ensuring reproducibility.
A final word
The challenges of scaling AI in medical imaging are multifaceted but can be overcome with a proactive approach. By honing in on the difficulties inherent in reader workflows and addressing them head-on, pharmaceutical giants and medical research institutions can ensure they’re not merely amassing colossal data lakes but effectively creating structured, organized data to advance patient care and medical research.
Streamlining imaging research is a mission beyond mere efficiency; it’s about unlocking the vast potential of AI and ML in medical imaging. Transitioning from cumbersome, home-grown methods to fluid, scalable mechanisms is within reach, and with it comes a future of unparalleled medical insights and breakthroughs.
Mark Mooney is the director of solutions marketing, pharma at Flywheel, a medical imaging AI development platform.